Math 10 Final Project: Analyzing the weather conditions in 10 locations#
Author: Zidong Zhang
Course Project, UC Irvine, Math 10, S24
I would like to post my notebook on the course’s website. [Yes]
import pandas as pd
import seaborn as sns
import altair as alt
import numpy as np
from sklearn.linear_model import LogisticRegression,LinearRegression
from sklearn.model_selection import train_test_split,KFold
from sklearn.metrics import r2_score
df = pd.read_csv('weather_data.csv')
print(df)
Location Date_Time Temperature_C Humidity_pct \
0 San Diego 2024/1/14 21:12 10.683001 41.195754
1 San Diego 2024/5/17 15:22 8.734140 58.319107
2 San Diego 2024/5/11 9:30 11.632436 38.820175
3 Philadelphia 2024/2/26 17:32 -8.628976 54.074474
4 San Antonio 2024/4/29 13:23 39.808213 72.899908
... ... ... ... ...
999995 Dallas 2024/1/1 20:29 23.416877 37.705024
999996 San Antonio 2024/1/20 15:59 6.759080 40.731036
999997 New York 2024/4/14 8:30 15.664465 62.201884
999998 Chicago 2024/5/12 20:10 18.999994 63.703245
999999 New York 2024/4/16 16:11 10.725351 43.804584
Precipitation_mm Wind_Speed_kmh
0 4.020119 8.233540
1 9.111623 27.715161
2 4.607511 28.732951
3 3.183720 26.367303
4 9.598282 29.898622
... ... ...
999995 3.819833 16.538119
999996 8.182785 29.005558
999997 3.987558 0.403909
999998 4.294325 6.326036
999999 1.883292 15.363828
[1000000 rows x 6 columns]
type(df)
pandas.core.frame.DataFrame
df.dtypes
Location object
Date_Time object
Temperature_C float64
Humidity_pct float64
Precipitation_mm float64
Wind_Speed_kmh float64
dtype: object
df.shape
(1000000, 6)
col =df['Location']
print(col)
type(col)
0 San Diego
1 San Diego
2 San Diego
3 Philadelphia
4 San Antonio
...
999995 Dallas
999996 San Antonio
999997 New York
999998 Chicago
999999 New York
Name: Location, Length: 1000000, dtype: object
pandas.core.series.Series
df.Humidity_pct.mean()
60.02182955554013
z = df[0:50]
z
Location | Date_Time | Temperature_C | Humidity_pct | Precipitation_mm | Wind_Speed_kmh | |
---|---|---|---|---|---|---|
0 | San Diego | 2024/1/14 21:12 | 10.683001 | 41.195754 | 4.020119 | 8.233540 |
1 | San Diego | 2024/5/17 15:22 | 8.734140 | 58.319107 | 9.111623 | 27.715161 |
2 | San Diego | 2024/5/11 9:30 | 11.632436 | 38.820175 | 4.607511 | 28.732951 |
3 | Philadelphia | 2024/2/26 17:32 | -8.628976 | 54.074474 | 3.183720 | 26.367303 |
4 | San Antonio | 2024/4/29 13:23 | 39.808213 | 72.899908 | 9.598282 | 29.898622 |
5 | San Diego | 2024/1/21 8:54 | 27.341055 | 49.023236 | 9.166543 | 27.473896 |
6 | San Jose | 2024/1/13 2:10 | 1.881883 | 65.742325 | 0.221709 | 1.073112 |
7 | New York | 2024/1/25 19:04 | -6.894766 | 30.804894 | 8.027624 | 16.848337 |
8 | New York | 2024/3/29 5:20 | 0.963545 | 38.819158 | 3.640129 | 7.989024 |
9 | San Jose | 2024/5/18 9:14 | -1.607088 | 82.198701 | 4.101493 | 25.647282 |
10 | New York | 2024/3/4 13:47 | 35.145559 | 54.752866 | 8.349195 | 25.430310 |
11 | Houston | 2024/3/7 22:03 | 15.816764 | 80.119902 | 3.760004 | 16.752132 |
12 | Dallas | 2024/2/27 21:07 | 32.016898 | 53.194371 | 3.552671 | 3.050196 |
13 | Houston | 2024/5/9 0:53 | 38.641269 | 85.952726 | 0.470782 | 20.779264 |
14 | Houston | 2024/5/12 15:57 | 39.666772 | 72.747026 | 1.263722 | 6.479492 |
15 | Philadelphia | 2024/3/9 1:51 | 28.290115 | 35.239170 | 9.347205 | 14.066765 |
16 | San Antonio | 2024/2/10 15:05 | 16.349790 | 65.812607 | 0.109090 | 6.597039 |
17 | Chicago | 2024/1/6 2:59 | 26.786811 | 31.513614 | 0.496024 | 22.980095 |
18 | San Antonio | 2024/5/8 16:20 | 35.179548 | 35.083071 | 9.597294 | 4.507863 |
19 | San Diego | 2024/1/31 5:38 | 14.605819 | 66.642235 | 1.515637 | 29.431890 |
20 | San Diego | 2024/1/25 12:59 | 33.023351 | 52.607485 | 0.212143 | 16.733325 |
21 | New York | 2024/2/19 12:26 | -7.383811 | 54.089973 | 1.905731 | 6.637064 |
22 | San Antonio | 2024/2/14 4:43 | 30.739684 | 85.603779 | 9.250559 | 24.375952 |
23 | San Jose | 2024/4/22 3:13 | 34.539654 | 57.793010 | 3.583560 | 13.044745 |
24 | Houston | 2024/1/11 2:53 | -5.236300 | 58.054296 | 4.072880 | 24.754053 |
25 | San Jose | 2024/3/5 21:38 | -1.733200 | 47.973206 | 7.066381 | 3.430741 |
26 | San Diego | 2024/3/27 10:44 | 24.312724 | 57.869279 | 9.693766 | 23.947188 |
27 | San Antonio | 2024/2/8 20:45 | 3.017951 | 49.868218 | 6.906614 | 6.212008 |
28 | New York | 2024/1/4 21:41 | 14.568328 | 41.350180 | 3.342765 | 4.781286 |
29 | Los Angeles | 2024/2/15 20:47 | -2.409511 | 46.834004 | 4.550643 | 7.437357 |
30 | New York | 2024/2/2 2:17 | -2.862063 | 53.395172 | 5.473387 | 28.519430 |
31 | Dallas | 2024/2/22 14:16 | 12.865779 | 44.725912 | 4.809865 | 5.567550 |
32 | Chicago | 2024/4/16 0:07 | 17.587820 | 32.817923 | 0.128803 | 0.234146 |
33 | Houston | 2024/2/29 23:17 | 7.630377 | 33.523611 | 2.462748 | 13.232696 |
34 | Philadelphia | 2024/4/20 9:34 | 0.351373 | 88.015157 | 1.795374 | 21.665466 |
35 | Dallas | 2024/3/22 2:34 | 17.439495 | 56.210161 | 9.728971 | 9.497027 |
36 | Philadelphia | 2024/3/16 23:47 | 23.405681 | 78.914506 | 7.767704 | 3.675792 |
37 | Phoenix | 2024/1/29 7:08 | 1.512627 | 89.417846 | 5.210117 | 7.332915 |
38 | Chicago | 2024/4/1 3:12 | -2.562660 | 30.356593 | 2.624328 | 2.601357 |
39 | New York | 2024/4/21 10:17 | 8.863086 | 71.492544 | 4.878107 | 21.904186 |
40 | Dallas | 2024/4/3 22:07 | 6.514284 | 42.006015 | 1.405197 | 18.385767 |
41 | New York | 2024/2/19 19:53 | 38.545534 | 40.450830 | 1.123494 | 13.262020 |
42 | Philadelphia | 2024/5/18 2:32 | -2.015243 | 36.062193 | 6.664136 | 18.623465 |
43 | San Diego | 2024/1/24 12:04 | -6.853765 | 84.666780 | 9.027691 | 18.233632 |
44 | San Diego | 2024/3/3 11:02 | 35.666565 | 74.060956 | 1.328726 | 24.161295 |
45 | Chicago | 2024/4/3 12:07 | 7.166150 | 50.377273 | 4.669553 | 11.841165 |
46 | Phoenix | 2024/3/24 22:44 | 34.453648 | 59.576973 | 4.514323 | 20.487150 |
47 | Chicago | 2024/4/5 13:22 | 38.386233 | 74.049712 | 6.792913 | 3.292467 |
48 | Philadelphia | 2024/3/26 12:45 | 7.106603 | 79.177323 | 5.767120 | 22.364479 |
49 | San Jose | 2024/5/8 20:16 | 35.097440 | 40.225820 | 6.088406 | 20.013380 |
df[0:2]
Location | Date_Time | Temperature_C | Humidity_pct | Precipitation_mm | Wind_Speed_kmh | |
---|---|---|---|---|---|---|
0 | San Diego | 2024/1/14 21:12 | 10.683001 | 41.195754 | 4.020119 | 8.233540 |
1 | San Diego | 2024/5/17 15:22 | 8.734140 | 58.319107 | 9.111623 | 27.715161 |
df[df['Location']== 'New York']
Location | Date_Time | Temperature_C | Humidity_pct | Precipitation_mm | Wind_Speed_kmh | |
---|---|---|---|---|---|---|
7 | New York | 2024/1/25 19:04 | -6.894766 | 30.804894 | 8.027624 | 16.848337 |
8 | New York | 2024/3/29 5:20 | 0.963545 | 38.819158 | 3.640129 | 7.989024 |
10 | New York | 2024/3/4 13:47 | 35.145559 | 54.752866 | 8.349195 | 25.430310 |
21 | New York | 2024/2/19 12:26 | -7.383811 | 54.089973 | 1.905731 | 6.637064 |
28 | New York | 2024/1/4 21:41 | 14.568328 | 41.350180 | 3.342765 | 4.781286 |
... | ... | ... | ... | ... | ... | ... |
999979 | New York | 2024/1/30 15:04 | 1.837912 | 66.505813 | 4.934130 | 4.797305 |
999990 | New York | 2024/1/1 11:49 | 20.245916 | 31.677558 | 9.801482 | 24.029331 |
999991 | New York | 2024/2/14 3:55 | 4.210758 | 45.683075 | 2.053384 | 22.351735 |
999997 | New York | 2024/4/14 8:30 | 15.664465 | 62.201884 | 3.987558 | 0.403909 |
999999 | New York | 2024/4/16 16:11 | 10.725351 | 43.804584 | 1.883292 | 15.363828 |
99972 rows × 6 columns
df.loc[0:50,['Location','Temperature_C']]
Location | Temperature_C | |
---|---|---|
0 | San Diego | 10.683001 |
1 | San Diego | 8.734140 |
2 | San Diego | 11.632436 |
3 | Philadelphia | -8.628976 |
4 | San Antonio | 39.808213 |
5 | San Diego | 27.341055 |
6 | San Jose | 1.881883 |
7 | New York | -6.894766 |
8 | New York | 0.963545 |
9 | San Jose | -1.607088 |
10 | New York | 35.145559 |
11 | Houston | 15.816764 |
12 | Dallas | 32.016898 |
13 | Houston | 38.641269 |
14 | Houston | 39.666772 |
15 | Philadelphia | 28.290115 |
16 | San Antonio | 16.349790 |
17 | Chicago | 26.786811 |
18 | San Antonio | 35.179548 |
19 | San Diego | 14.605819 |
20 | San Diego | 33.023351 |
21 | New York | -7.383811 |
22 | San Antonio | 30.739684 |
23 | San Jose | 34.539654 |
24 | Houston | -5.236300 |
25 | San Jose | -1.733200 |
26 | San Diego | 24.312724 |
27 | San Antonio | 3.017951 |
28 | New York | 14.568328 |
29 | Los Angeles | -2.409511 |
30 | New York | -2.862063 |
31 | Dallas | 12.865779 |
32 | Chicago | 17.587820 |
33 | Houston | 7.630377 |
34 | Philadelphia | 0.351373 |
35 | Dallas | 17.439495 |
36 | Philadelphia | 23.405681 |
37 | Phoenix | 1.512627 |
38 | Chicago | -2.562660 |
39 | New York | 8.863086 |
40 | Dallas | 6.514284 |
41 | New York | 38.545534 |
42 | Philadelphia | -2.015243 |
43 | San Diego | -6.853765 |
44 | San Diego | 35.666565 |
45 | Chicago | 7.166150 |
46 | Phoenix | 34.453648 |
47 | Chicago | 38.386233 |
48 | Philadelphia | 7.106603 |
49 | San Jose | 35.097440 |
50 | San Diego | -5.855824 |
df.loc[:30,'Humidity_pct':'Wind_Speed_kmh']
Humidity_pct | Precipitation_mm | Wind_Speed_kmh | |
---|---|---|---|
0 | 41.195754 | 4.020119 | 8.233540 |
1 | 58.319107 | 9.111623 | 27.715161 |
2 | 38.820175 | 4.607511 | 28.732951 |
3 | 54.074474 | 3.183720 | 26.367303 |
4 | 72.899908 | 9.598282 | 29.898622 |
5 | 49.023236 | 9.166543 | 27.473896 |
6 | 65.742325 | 0.221709 | 1.073112 |
7 | 30.804894 | 8.027624 | 16.848337 |
8 | 38.819158 | 3.640129 | 7.989024 |
9 | 82.198701 | 4.101493 | 25.647282 |
10 | 54.752866 | 8.349195 | 25.430310 |
11 | 80.119902 | 3.760004 | 16.752132 |
12 | 53.194371 | 3.552671 | 3.050196 |
13 | 85.952726 | 0.470782 | 20.779264 |
14 | 72.747026 | 1.263722 | 6.479492 |
15 | 35.239170 | 9.347205 | 14.066765 |
16 | 65.812607 | 0.109090 | 6.597039 |
17 | 31.513614 | 0.496024 | 22.980095 |
18 | 35.083071 | 9.597294 | 4.507863 |
19 | 66.642235 | 1.515637 | 29.431890 |
20 | 52.607485 | 0.212143 | 16.733325 |
21 | 54.089973 | 1.905731 | 6.637064 |
22 | 85.603779 | 9.250559 | 24.375952 |
23 | 57.793010 | 3.583560 | 13.044745 |
24 | 58.054296 | 4.072880 | 24.754053 |
25 | 47.973206 | 7.066381 | 3.430741 |
26 | 57.869279 | 9.693766 | 23.947188 |
27 | 49.868218 | 6.906614 | 6.212008 |
28 | 41.350180 | 3.342765 | 4.781286 |
29 | 46.834004 | 4.550643 | 7.437357 |
30 | 53.395172 | 5.473387 | 28.519430 |
df.iloc[0:3,0:3]
Location | Date_Time | Temperature_C | |
---|---|---|---|
0 | San Diego | 2024/1/14 21:12 | 10.683001 |
1 | San Diego | 2024/5/17 15:22 | 8.734140 |
2 | San Diego | 2024/5/11 9:30 | 11.632436 |
df.describe()
Temperature_C | Humidity_pct | Precipitation_mm | Wind_Speed_kmh | |
---|---|---|---|---|
count | 1000000.000000 | 1000000.000000 | 1000000.000000 | 1000000.000000 |
mean | 14.779705 | 60.021830 | 5.109639 | 14.997598 |
std | 14.482558 | 17.324022 | 2.947997 | 8.663556 |
min | -19.969311 | 30.000009 | 0.000009 | 0.000051 |
25% | 2.269631 | 45.008500 | 2.580694 | 7.490101 |
50% | 14.778002 | 60.018708 | 5.109917 | 14.993777 |
75% | 27.270489 | 75.043818 | 7.613750 | 22.514110 |
max | 39.999801 | 89.999977 | 14.971583 | 29.999973 |
df.info()
<class 'pandas.core.frame.DataFrame'>
RangeIndex: 1000000 entries, 0 to 999999
Data columns (total 6 columns):
# Column Non-Null Count Dtype
--- ------ -------------- -----
0 Location 1000000 non-null object
1 Date_Time 1000000 non-null object
2 Temperature_C 1000000 non-null float64
3 Humidity_pct 1000000 non-null float64
4 Precipitation_mm 1000000 non-null float64
5 Wind_Speed_kmh 1000000 non-null float64
dtypes: float64(4), object(2)
memory usage: 45.8+ MB
a =df.dropna(thresh=6)
a
Location | Date_Time | Temperature_C | Humidity_pct | Precipitation_mm | Wind_Speed_kmh | |
---|---|---|---|---|---|---|
0 | San Diego | 2024/1/14 21:12 | 10.683001 | 41.195754 | 4.020119 | 8.233540 |
1 | San Diego | 2024/5/17 15:22 | 8.734140 | 58.319107 | 9.111623 | 27.715161 |
2 | San Diego | 2024/5/11 9:30 | 11.632436 | 38.820175 | 4.607511 | 28.732951 |
3 | Philadelphia | 2024/2/26 17:32 | -8.628976 | 54.074474 | 3.183720 | 26.367303 |
4 | San Antonio | 2024/4/29 13:23 | 39.808213 | 72.899908 | 9.598282 | 29.898622 |
... | ... | ... | ... | ... | ... | ... |
999995 | Dallas | 2024/1/1 20:29 | 23.416877 | 37.705024 | 3.819833 | 16.538119 |
999996 | San Antonio | 2024/1/20 15:59 | 6.759080 | 40.731036 | 8.182785 | 29.005558 |
999997 | New York | 2024/4/14 8:30 | 15.664465 | 62.201884 | 3.987558 | 0.403909 |
999998 | Chicago | 2024/5/12 20:10 | 18.999994 | 63.703245 | 4.294325 | 6.326036 |
999999 | New York | 2024/4/16 16:11 | 10.725351 | 43.804584 | 1.883292 | 15.363828 |
1000000 rows × 6 columns
top_five = df['Location'].value_counts().head(5)
top = top_five.index.values
top
array(['Phoenix', 'Chicago', 'Philadelphia', 'Houston', 'New York'],
dtype=object)
cf = df[df['Location'].isin(top)]
cf
Location | Date_Time | Temperature_C | Humidity_pct | Precipitation_mm | Wind_Speed_kmh | |
---|---|---|---|---|---|---|
3 | Philadelphia | 2024/2/26 17:32 | -8.628976 | 54.074474 | 3.183720 | 26.367303 |
7 | New York | 2024/1/25 19:04 | -6.894766 | 30.804894 | 8.027624 | 16.848337 |
8 | New York | 2024/3/29 5:20 | 0.963545 | 38.819158 | 3.640129 | 7.989024 |
10 | New York | 2024/3/4 13:47 | 35.145559 | 54.752866 | 8.349195 | 25.430310 |
11 | Houston | 2024/3/7 22:03 | 15.816764 | 80.119902 | 3.760004 | 16.752132 |
... | ... | ... | ... | ... | ... | ... |
999990 | New York | 2024/1/1 11:49 | 20.245916 | 31.677558 | 9.801482 | 24.029331 |
999991 | New York | 2024/2/14 3:55 | 4.210758 | 45.683075 | 2.053384 | 22.351735 |
999997 | New York | 2024/4/14 8:30 | 15.664465 | 62.201884 | 3.987558 | 0.403909 |
999998 | Chicago | 2024/5/12 20:10 | 18.999994 | 63.703245 | 4.294325 | 6.326036 |
999999 | New York | 2024/4/16 16:11 | 10.725351 | 43.804584 | 1.883292 | 15.363828 |
500543 rows × 6 columns
alt.data_transformers.disable_max_rows()
DataTransformerRegistry.enable('default')
columns = ['Temperature_C','Humidity_pct','Precipitation_mm','Wind_Speed_kmh']
d1 = alt.Chart(a[0:1000]).mark_bar().encode(x ='Location',y ='Humidity_pct',color = 'Location')
d2 = alt.Chart(cf[0:1000]).mark_point().encode(x ='Temperature_C',y ='Humidity_pct',color = 'Location')
d3 = alt.Chart(cf[0:1000]).mark_point().encode(x ='Temperature_C',y ='Precipitation_mm',color = 'Location')
d4 = alt.Chart(cf[0:1000]).mark_point().encode(x ='Temperature_C',y ='Wind_Speed_kmh',color = 'Location')
d1|d2|d3|d4
The picture shows that from the first 1000 data and analyze, it shows the bar charta and point chart. It shwos that the relationship between the temperature and humidity, precipitaion and wind speed. We can see that Chicago and New York are moister than other location.
Logistic Regression#
lg = LogisticRegression()
lg.fit(cf[columns],cf['Location'])
LogisticRegression()In a Jupyter environment, please rerun this cell to show the HTML representation or trust the notebook.
On GitHub, the HTML representation is unable to render, please try loading this page with nbviewer.org.
LogisticRegression()
lg.predict(cf[columns])
array(['Houston', 'Phoenix', 'Houston', ..., 'New York', 'Philadelphia',
'Houston'], dtype=object)
lg.score(cf[columns],cf['Location'])
0.22513350501355528
lg.coef_
array([[ 2.06782623e-03, 1.62776332e-04, -2.39521432e-02,
1.23933356e-04],
[ 1.74647013e-03, -2.58646999e-04, -2.46208594e-02,
3.75893962e-07],
[ 2.08240756e-03, 8.82963083e-06, -2.51033553e-02,
5.98420218e-06],
[ 2.19137751e-03, 1.58940926e-05, -2.33413604e-02,
1.04776813e-05],
[-8.08808143e-03, 7.11469430e-05, 9.70177183e-02,
-1.40771133e-04]])
lg.classes_
array(['Chicago', 'Houston', 'New York', 'Philadelphia', 'Phoenix'],
dtype=object)
lg.intercept_
array([ 0.0930484 , 0.12760107, 0.10777946, 0.09835607, -0.42678501])
I use code to calculate the logistic Regression Coefficients, intercepts and the score.
Q = d1.mark_bar().encode()
print(Q)
alt.Chart(...)
Q
Cross Validation#
features = ['Precipitation_mm']
target = ['Wind_Speed_kmh']
df.dropna(subset=features + target, inplace=True)
model = LinearRegression()
kf =KFold(n_splits=5,shuffle=True,random_state=1)
scores = []
k = 1
for train_index, test_index in kf.split(df):
X_train, X_test = df[features].iloc[train_index], df[features].iloc[test_index]
y_train, y_test = df[target].iloc[train_index], df[target].iloc[test_index]
model.fit(X_train,y_train)
y_pred = model.predict(X_test)
score = r2_score(y_test,y_pred)
scores.append(score)
k += 1
print(f"Fold {k-1} R^2 score:",score)
print('Average R^2 score:',np.mean(scores))
Fold 1 R^2 score: 1.945889039789428e-06
Fold 2 R^2 score: 1.5445602918884305e-07
Fold 3 R^2 score: -5.1999608152719645e-06
Fold 4 R^2 score: -1.3250731167646634e-05
Fold 5 R^2 score: -1.3370042832638873e-05
Average R^2 score: -5.94407794931584e-06
In this case, I calculate the Fold 1,2,3,4,5 and use cross validation to find the mean scores.
from sklearn.preprocessing import StandardScaler
from sklearn.preprocessing import LabelEncoder
features = ['Precipitation_mm','Wind_Speed_kmh']
label = 'Location'
df.dropna(subset=features + [label], inplace=True)
scaler = StandardScaler()
df[features] = scaler.fit_transform(df[features])
X= df[features]
y= df[label]
label_encoder = LabelEncoder()
df[label] = label_encoder.fit_transform(df[label])
X = df[features]
y = df[label]
import numpy as np
import pandas as pd
import matplotlib.pyplot as plt
from sklearn.linear_model import LogisticRegression
from sklearn.metrics import accuracy_score
model = LogisticRegression(multi_class='multinomial', solver='lbfgs')
model.fit(X,y)
y_pred = model.predict(X)
accuracy = accuracy_score(y,y_pred)
print('Accuracy:',accuracy)
print(y_pred)
Accuracy: 0.111441
[9 6 3 ... 7 7 9]
plt.figure(figsize=(10,10))
sns.barplot(x='Humidity_pct',y='Temperature_C',data=df[0:100],errorbar= None,edgecolor= 'black')
plt.title('The data between Temperature and Humidity')
print()
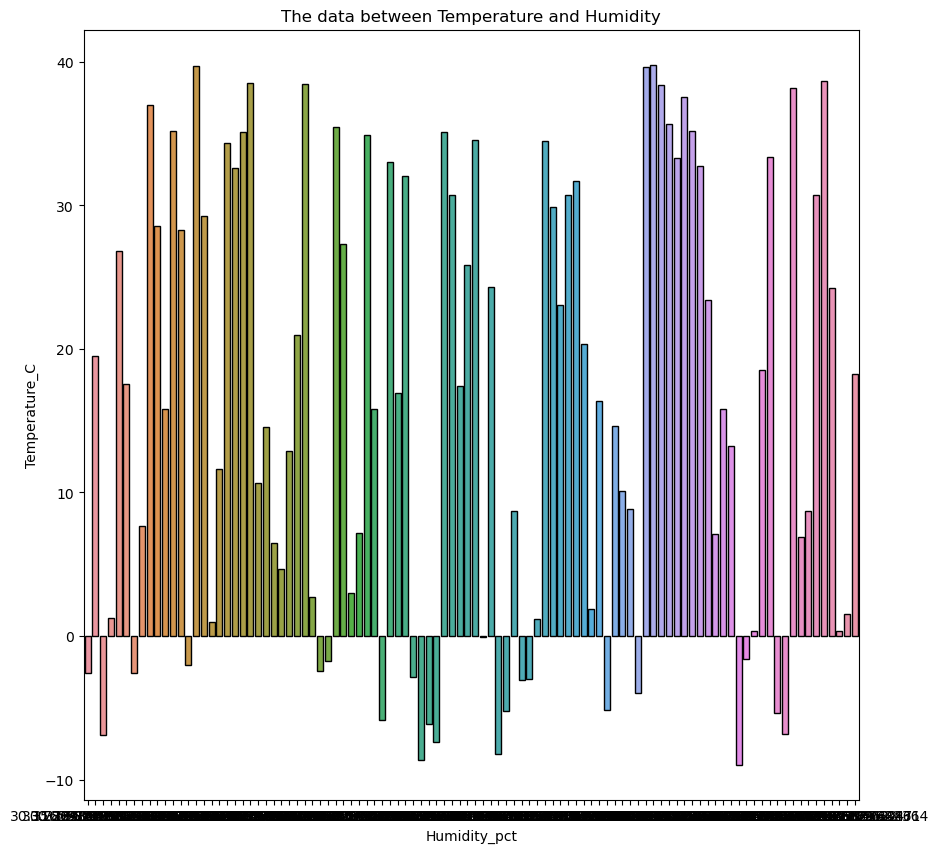
Extra: using Naive Bayes Classifier and compare the data#
from sklearn.naive_bayes import GaussianNB
X_train, X_test, y_train, y_test = train_test_split(X,y,test_size=0.2,random_state=1,stratify=y)
model_1 = GaussianNB()
model_1.fit(X_train,y_train)
GaussianNB()In a Jupyter environment, please rerun this cell to show the HTML representation or trust the notebook.
On GitHub, the HTML representation is unable to render, please try loading this page with nbviewer.org.
GaussianNB()
model_1.score(X_test,y_test)
0.111875
y_p = model_1.predict(X_test)
print(y_p)
[7 1 7 ... 7 6 6]
min(y_p)
0
max(y_p)
9
m = np.mean(y_p)
print(m)
4.287705
f1 = np.std(y_p)
print(f1)
2.546544881398127
accuracy = accuracy_score(y_test,y_p)
print(accuracy)
0.111875
model_1.fit(X,y)
GaussianNB()In a Jupyter environment, please rerun this cell to show the HTML representation or trust the notebook.
On GitHub, the HTML representation is unable to render, please try loading this page with nbviewer.org.
GaussianNB()
model_1.score(X,y)
0.111734
y_n = model_1.predict(X)
print(y_n)
[9 6 8 ... 7 7 9]
accuracy_n = accuracy_score(y,y_n)
print(accuracy_n)
0.111734
max(y_n)
9
min(y_n)
0
n = np.mean(y_n)
print(n)
5.848463
f = np.std(y_n)
print(f)
2.7098670701034395
max(m,n)
5.848463
max(f1,f)
2.7098670701034395
Reference#
This is the website: https://www.kaggle.com/datasets/prasad22/weather-data
Some resources about Naive Bayes Classifer: https://www.geeksforgeeks.org/naive-bayes-classifiers/